
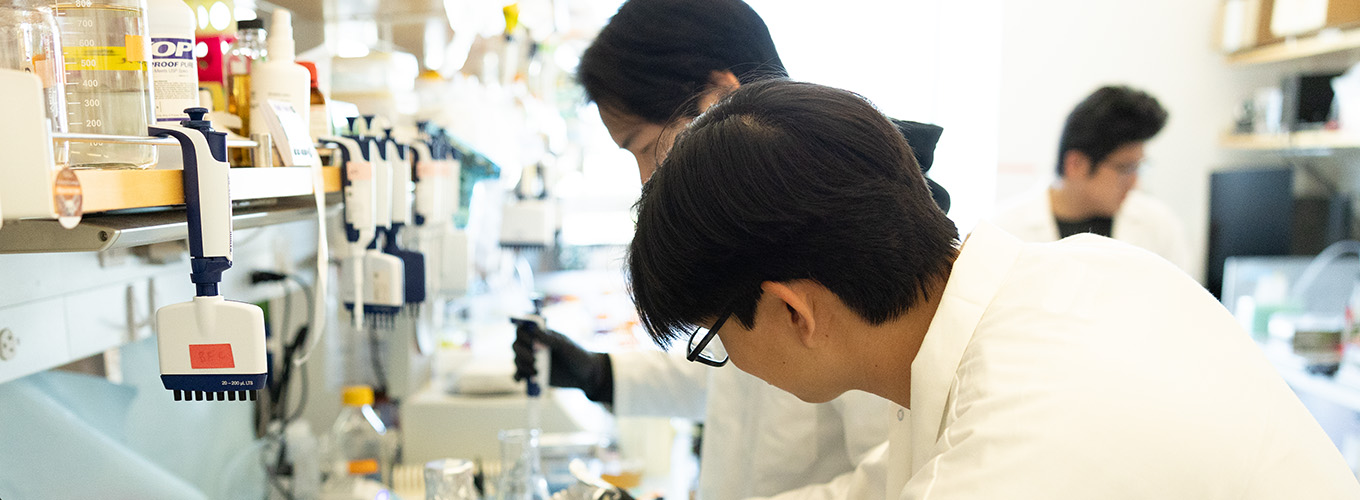
Combining AI-based protein structures from AlphaFold2 with older computational tools was key to finding previously unidentified Cas13 enzymes.
Since the discovery of CRISPR-Cas9, Jennifer Doudna’s lab and others around the world have been studying the evolution of CRISPR systems and trying to discover new ones. In a new paper in Science out today, Doudna and colleagues uncover the history of a unique CRISPR-Cas system and describe a new method of using machine learning tools to uncover new enzymes.
Scientists looking to discover different CRISPR-Cas systems in nature typically mine genomic sequence databases looking for homology, that is proteins that have highly similar amino acid sequences. This approach has led to discovery of new genome-editing tools with novel characteristics including smaller size for easier delivery into cells and different properties that have been developed into new technologies.
Over time, researchers started to find homology between not just CRISPR proteins, but other proteins as well. In the case of the two best-known CRISPR associated enzymes, Cas9 and Cas12, researchers found that they shared similarities with genes encoded in transposons, the parts of a genome also known as ”jumping genes” that can change their location.
“The sequence homology was clear and noticed very early on,” says Peter Yoon, first author on the new paper. “When people began to look into the function of those enzymes, they realized that they act very similarly to Cas9 and Cas12, where they use an RNA guide to cut double-stranded DNA.”
While the transposon-encoded genes don’t function as defense against viruses like CRISPR-Cas systems, the two use a similar underlying mechanism to achieve different tasks.
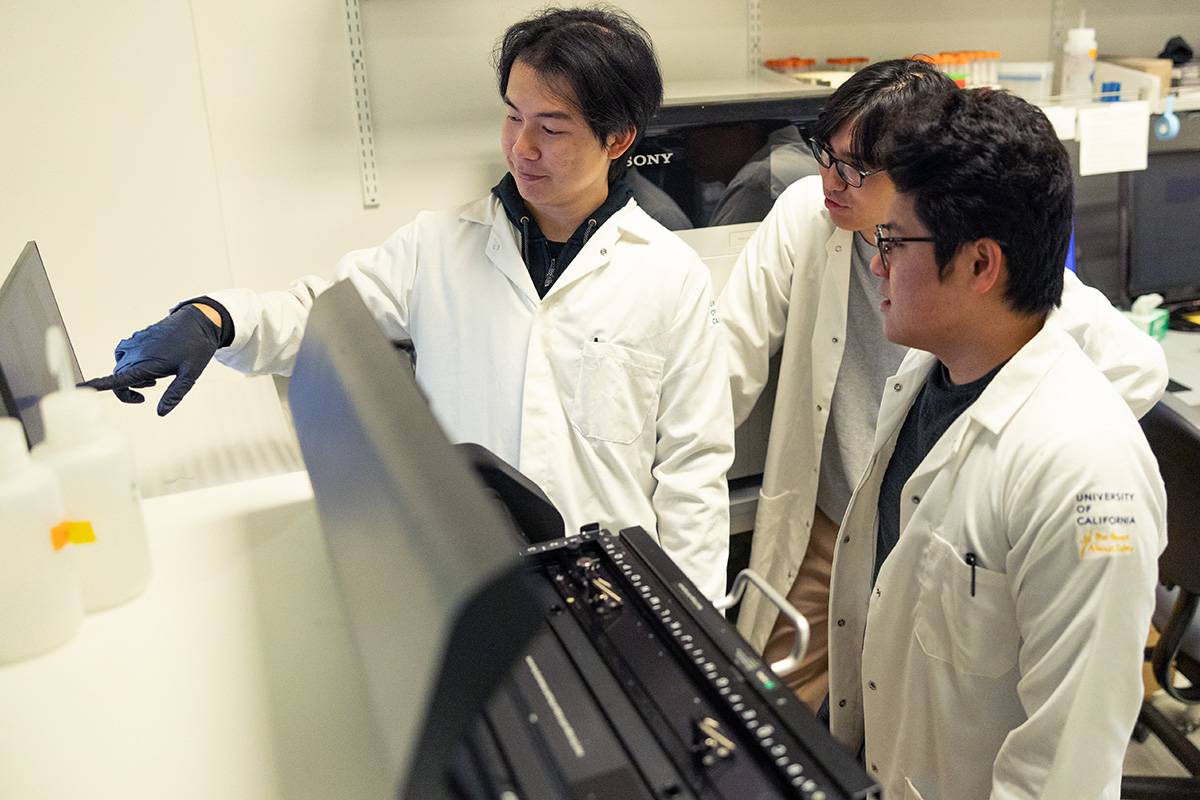
Yoon was interested in looking at another CRISPR enzyme, Cas13, which unlike Cas9 and Cas12 cuts RNA not DNA. Its evolutionary history has been less clear. The barrier for researchers has been that the tools used to reconstruct the history of the other Cas enzymes aren’t helpful with Cas13.
“The sequence similarity between given Cas13 proteins is very low. So if you have a sequence of one Cas13 protein, you’re going to have a hard time finding another,” says Yoon.
Because of this, sequence databases haven’t been useful. But while the sequences change significantly between the known Cas13 enzymes, the overall shape or structure of the molecules remains quite similar.
In recent years, with the help of AI and machine learning tools like AlphaFold2, structural databases have been exploding. This gave Yoon hope that there would be a way to find other Cas13s by searching for similar structures regardless of the amino acid sequence.
“The most popular program these days is called Foldseek, a machine learning program that is supposed to have comparable performance to the traditional structural comparison programs that were really accurate and considered gold standard, but orders of magnitude faster,” says Yoon.
In this particular case “comparable performance” wasn’t quite comparable enough.
“When you go to the extreme remote homology that we are interested in understanding — that is, where does Cas13 come from? — the slight difference in sensitivity can make the difference between finding something and not finding anything at all,” says Yoon.
The team was faced with a machine learning tool that was fast and could handle huge data sets but wasn’t sensitive enough, or an older structural comparison tool that was highly sensitive but couldn’t handle an analysis the size of the over 200 million structures in the AlphaFold database. The answer turned out to be a clever combination of both tools.
Based on a recent paper that showed that the machine learning tool could also be used to cluster similar structures, bringing down the search space 100-fold to around 2.3 million structures. However, even this was not small enough for the slower but more accurate program to handle. To overcome this, Yoon and his colleagues ran multiple analyses in parallel on the Wynton High-Performance Compute Cluster at UCSF, reducing the search time from six months to a matter of minutes. With this new analysis, the team was able to discover Cas13 proteins that had never been found before, some of which were remarkably different from known proteins.
“The typical size of a Cas13 protein, the smallest ones found are around 800 amino acids, and the largest ones are around 1400 amino acids. The ones we found were around 450 amino acids — half the size, or even a third the size of the larger ones,” says Yoon.
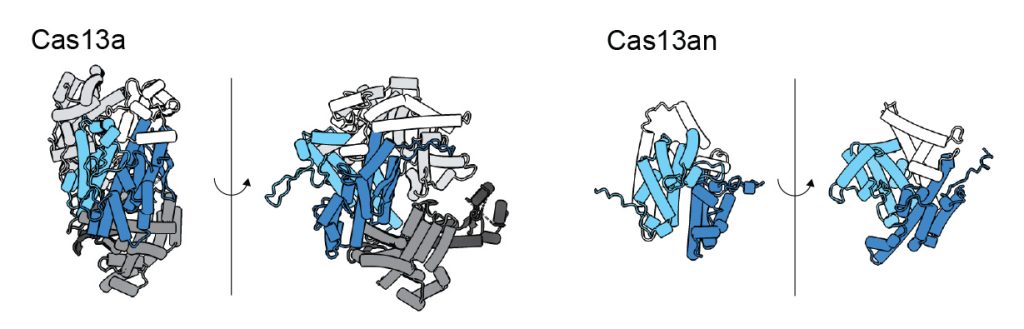
The authors used this newly discovered protein as a starting point to uncover the origins of Cas13, and found that their newly identified small enzyme represents the “ancestral state” of Cas13s. They also found that Cas13 enzymes are most closely related to a group of other enzymes involved in bacterial defense and associated with a non-coding RNA, suggesting these features may have come before Cas13’s CRISPR association.
As with Cas9 and Cas12, earlier diverging Cas13 enzymes are smaller and simpler, some of them containing just the nuclease, i.e., the RNA-cutting region, and little else. These small Cas13 enzymes are still fully functional, expanding the RNA manipulation toolkit for researchers.
“It’s really interesting to see that within the different CRISPR systems, we see recurring patterns in their evolutionary histories. So despite being unrelated, the same thing was happening independently in parallel. Personally, that’s a really fascinating story,” says Yoon.
It’s also a story that could not have been told without a productive combination of old and new tools, where the whole turned out to be greater than the sum of its parts. AI and machine learning didn’t replace the older tool, but expanded the possibilities of what it could do.
“In many organisms from bacteria to humans, 40–80 percent of the genes encode proteins of unknown function,” says Doudna. “This study shows how such protein functions can be discovered by comparative analysis of AI-generated structure databases like AlphaFold. Our study answered fundamental questions about CRISPR evolution and uncovered new genome-editing tools. And beyond these findings, our strategy can easily be applied to other exciting biological questions.”
The field of machine-learning based search tools is currently growing rapidly. Yoon is positive about where the field is already but also sees room for improvement. This study is a reminder that a close approximation is not always close enough for science. Yoon’s hope is that AI computational tools will advance to the point where additional specialized resources won’t have to be required to make new discoveries.
Read more:
Structure Guided Discovery of Ancestral CRISPR-Cas13 Ribonucleases. Peter H. Yoon, Zeyuan Zhang, Kenneth J. Loi, Benjamin A. Adler, Arushi Lahiri, Kamakshi Vohra, Honglue Shi, Daniel Bellieny Rabelo, Marena Trinidad, Ron S. Boger, Muntathar J. Al-Shimary, and Jennifer A. Doudna. July 18, 2024. Science
This research was supported by the National Science Foundation, the Howard Hughes Medical Institute, and m-CAFEs Microbial Community Analysis & Functional Evaluation in Soils at Lawrence Berkeley National Laboratory.